Modern Methods For Robust Regression Pdf To Word
1Department of Ecology and Evolutionary Biology, University of California Los Angeles, 610 Charles E. Young Drive East, Los Angeles, CA,, USA; 2Department of Paleobiology & Division of Mammals, National Museum of Natural History, Smithsonian Institution, MRC 121, PO Box 37012, Washington, DC.,, USA; 3Institute for Bioinformatics and Evolutionary Studies, University of Idaho, 441D Life Sciences South, PO Box 443051, Moscow, ID,, USA; and 4National Evolutionary Synthesis Center, 2024 W.
Oct 22, 2013. Second, we use a robust regression procedure that allows for the identification and down-weighting of convergent taxa, leading to moderate increases in method performance. We demonstrate the utility and power of these approach by investigating the evolution of body size in cetaceans. Model fitting using. In a way that is refreshingly engaging and readable, Wright and London describe the most useful regression techniques and provide step-by-step instructions.

Main Street, Suite A200, Durham, NC,, USA. 1Department of Ecology and Evolutionary Biology, University of California Los Angeles, 610 Charles E.
Young Drive East, Los Angeles, CA,, USA; 2Department of Paleobiology & Division of Mammals, National Museum of Natural History, Smithsonian Institution, MRC 121, PO Box 37012, Washington, DC.,, USA; 3Institute for Bioinformatics and Evolutionary Studies, University of Idaho, 441D Life Sciences South, PO Box 443051, Moscow, ID,, USA; and 4National Evolutionary Synthesis Center, 2024 W. Main Street, Suite A200, Durham, NC,, USA.
Abstract A central prediction of much theory on adaptive radiations is that traits should evolve rapidly during the early stages of a clade's history and subsequently slowdown in rate as niches become saturated—a so-called “Early Burst.” Although a common pattern in the fossil record, evidence for early bursts of trait evolution in phylogenetic comparative data has been equivocal at best. We show here that this may not necessarily be due to the absence of this pattern in nature. Rather, commonly used methods to infer its presence perform poorly when when the strength of the burst—the rate at which phenotypic evolution declines—is small, and when some morphological convergence is present within the clade. We present two modifications to existing comparative methods that allow greater power to detect early bursts in simulated datasets. First, we develop posterior predictive simulation approaches and show that they outperform maximum likelihood approaches at identifying early bursts at moderate strength. Second, we use a robust regression procedure that allows for the identification and down-weighting of convergent taxa, leading to moderate increases in method performance. We demonstrate the utility and power of these approach by investigating the evolution of body size in cetaceans.
Model fitting using maximum likelihood is equivocal with regards the mode of cetacean body size evolution. However, posterior predictive simulation combined with a robust node height test return low support for Brownian motion or rate shift models, but not the early burst model. While the jury is still out on whether early bursts are actually common in nature, our approach will hopefully facilitate more robust testing of this hypothesis. Crack Wpa2 Beini Software here. We advocate the adoption of similar posterior predictive approaches to improve the fit and to assess the adequacy of macroevolutionary models in general. [Adaptive Radiations, Early Burst, Posterior Predictive Simulations, Quantitative Characters].
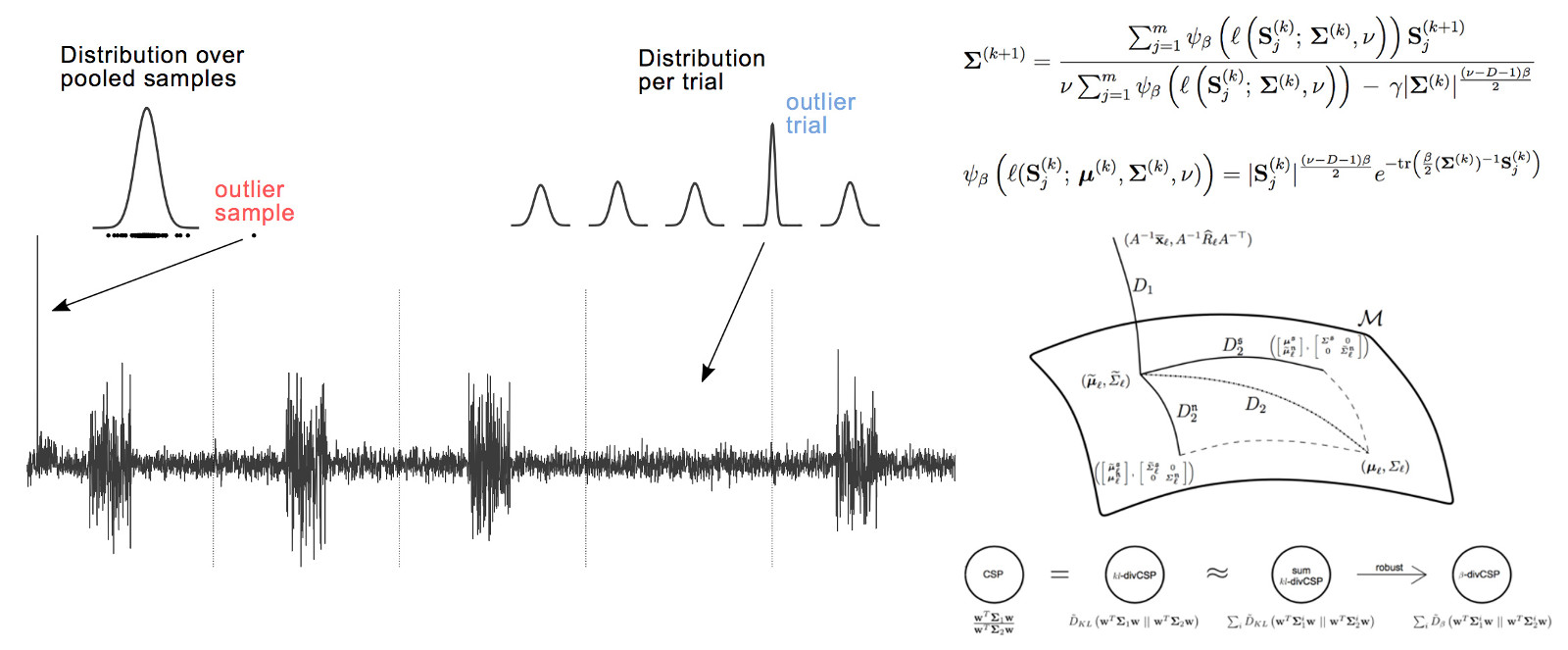
At the end of the Cretaceous, just before an asteroid and its aftermath wiped out huge swaths of life on earth including the dinosaurs, most mammals were small and, at least compared to today, unremarkable in their diversity (; ). Modern mammalian lineages, many of which had been in existence since the mid-Cretaceous, only subsequently diversified into the diversity of forms we see today (;;; ). Characterizing the rise of this diversity has long preoccupied evolutionary biologists, and an intriguing narrative emerges from the plethora of studies of the mammalian fossil record. While there have been successive waves of lineage diversification in mammals (), this appears not to be the case for many important ecomorphological characters.
Rather, disparity in such traits as dentition and body size peaked relatively early in the radiation of mammals and then stabilized (; ). The ecomorphological space available to mammals appears to have been saturated early in the Cenozoic, with subsequent lineages representing variations on a few themes. In a broad sense, this is what George Gaylord; had in mind when he wrote of adaptive radiations occurring within “adaptive zones.” The notion of adaptive radiations continues to intrigue evolutionary biologists today, and many agree with suggestion that these have generated much of the biodiversity on earth. While there has been a great deal of discussion as to exactly what constitutes an adaptive radiation (;;;;; ), a general expectation from much of this verbal theory is that character evolution should show a fairly stereotypical pattern through time. Under the scenario classically envisaged (,; ), rates of evolution should be rapid early in a clade's history as character displacement and other mechanisms drive species to diverge and should subsequently slow as niches fill up and competition with incumbent species prevents further divergence. This pattern should leave a signature on the distribution of trait values at the tips of a phylogeny (;,; ) and thus in principle be detectable by phylogenetic comparative methods. Although the early burst pattern of phenotypic evolution has received much support in paleontological studies (,,,;;; ), evidence for its pervasiveness in phylogenetic comparative datasets has been mixed at best.
Early, rapid trait evolution has been documented in ratsnakes (), Australian agamid lizards (), cetaceans (), ovenbirds (), and triggerfishes (). Used a model of diversity-dependent trait evolution to show that the Antillean Anolis radiation showed patterns consistent with niche-filling within each island assemblage.
In a broader comparative study, examined 49 clades including some of the most iconic adaptive radiations. Using a maximum likelihood approach (see later in the text), they found very little support for the Early Burst (EB) model across their datasets; most were better explained by either a Brownian motion (BM) or Ornstein-Uhlenbeck (OU) model of evolution. From this analysis, they suggested that the pattern of a slowdown in evolutionary rate often associated with adaptive radiations was actually rare in comparative data. An analysis of body size evolution across mammalian phylogeny similarly failed to recover evidence for early and rapid evolution, instead finding stronger support for branch-specific variation in evolutionary rates (; but see ). There are several reason to believe a lack of power, rather than a lack of generality, may underlie our ability to detect early bursts of evolution in phylogenetic comparative datasets.
First, for many small datasets, we lack sufficient power to reject simple models of evolution using information theoretic approaches (i.e., high Type II error rates; ). Of the 49 clades used by, 39 had n ≤ 40 taxa and 29 had n ≤ 20 taxa. It can easily be shown through simulation that early bursts of trait evolution are almost completely unidentifiable in clades of these sizes, even when evolution proceeds in exceptionally rapid bursts (). Second, we are often limited to testing for a time-dependent process using data from a single time-slice—the present day. Although the signature of an early burst should be retained in phylogenetic comparative datasets of extant species, power to detect this signature increases if extinct taxa are included ().
Finally, the EB model assumes that trait evolution slowed at the same pace across the entire clade. If trait evolution in some lineages strayed from the underlying process, perhaps due to convergent selection or an escape from the adaptive zone, then these secondary processes may deteriorate the power of the likelihood formulation of the EB model to detect an early burst. For example, found evidence for an early burst of body size evolution in cetaceans only after removing two secondarily large dolphin species, from their dataset. They attributed the deviation in body size evolution in these lineages to convergence resulting from ecological replacement of large predatory sperm whale lineages that went extinct in the late Miocene (e.g., ).
Such a pattern does not invalidate an overall pattern of declining rates resulting from niche filling sensu,; no theory of adaptive radiation explicitly requires monophyly and extinction of taxa or entire clades within an adaptively radiating lineage will create ecological opportunity that closely related lineages are expected to fill (; ). As the statistician George Box famously remarked, “all models are wrong, but some are useful.” The EB model as explicitly formulated is likely to be wrong (as are BM and OU). The question of whether it is useful for identifying early rapid evolution in comparative datasets remains to be answered.
Power to detect Early Bursts (EBs) of trait evolution in comparative datasets. We simulated early bursts of trait evolution under a range of exponential decline parameters on 10,000 simulated phylogenies containing between 10 and 200 extant taxa (resulting in a 20 × 11 grid with an average of 446 simulations per point). We then fit Brownian Motion (BM) and EB models to the simulated data and compared model fit using Akaike Weights. Mean weights for the EB model are plotted here as a contour plot, with light colors indicating low support and dark colors indicating high support for EB. EBs can only be detected with strong support from large trees with rapidly declining rates (see text for explanation of rate half-life). Note that because BM is a special case of EB, weights are expected to be >0 when the number of elapsed rate half lives is zero.
Power to detect Early Bursts (EBs) of trait evolution in comparative datasets. We simulated early bursts of trait evolution under a range of exponential decline parameters on 10,000 simulated phylogenies containing between 10 and 200 extant taxa (resulting in a 20 × 11 grid with an average of 446 simulations per point).
We then fit Brownian Motion (BM) and EB models to the simulated data and compared model fit using Akaike Weights. Mean weights for the EB model are plotted here as a contour plot, with light colors indicating low support and dark colors indicating high support for EB. EBs can only be detected with strong support from large trees with rapidly declining rates (see text for explanation of rate half-life). Note that because BM is a special case of EB, weights are expected to be >0 when the number of elapsed rate half lives is zero. Despite having great potential to aid in assessing model fit, predictive approaches have received little attention in the field of comparative methods in general, and for studying modes of trait evolution in particular (but see ). Posterior predictive approaches differ from traditional Bayesian methods in shifting the focus of model selection from choosing the model that maximizes the posterior probability of the observed data to choosing the model that best predicts the observed data via simulation (). Predictive approaches are best known to comparative biologists through the pioneering work of, whose innovative stochastic character mapping approach allowed for probabilistic inference of the locations of character state transitions on phylogenies (also see;;; ).
Simulation-based approaches have also been used to detect rate shifts (; ), non-Brownian modes of evolution () and to assess the adequacy of models of diversification (; ). Related methods have also been applied to selecting models of sequence evolution for inferring phylogenetic trees (e.g.,; ). These results raise the possibility that posterior predictive approaches might not only provide comparative biologists with a measure of model adequacy, but also with a powerful tool for comparing the fit of different models to trait data, particularly in cases where traditional likelihood approaches are known to have low power. In this article, we develop two posterior predictive approaches for detecting early bursts of trait evolution based on existing comparative methods. We then use simulations to evaluate their power relative to a more commonly used maximum likelihood approach. Perhaps surprisingly, we find that the maximum likelihood approach has somewhat lower power to detect early bursts than the two predictive approaches, particularly when the decline in rate is not incredibly strong. This difference is greatly exacerbated when even a moderate number of ‘outlier’ taxa (lineages that do not fit the ancestral evolutionary model) are introduced into the clade.
We present an additional modification to one of our predictive approaches that makes use of a robust regression procedure to reduce the influence of such outliers, and show that this improves our power to detect early bursts of trait evolution in simulated datasets. Finally, we demonstrate the utility of these approaches using a dataset of cetacean body lengths (), and show that our robust regression procedure provides little support for two alternative models of trait evolution while favoring the early burst hypothesis. Although we cannot claim anything from this study regarding the generality of the early burst pattern in nature, we do suggest that the evidence refuting its frequency is still equivocal and requires further attention. MATERIALS AND METHODS Methods for Detecting Early Bursts We begin by briefly reviewing the three commonly used approaches for detecting early bursts of trait evolution using phylogenetic comparative data: disparity through time analysis (), the node height test (), and maximum likelihood (). Disparity Through Time analysis (henceforth DTT; ) is similar in spirit to approaches used by paleobiologists (e.g., ) in that the method uses the average pairwise Euclidean distance between species as a measure of disparity.
Disparity is first computed for the entire clade, and subsequently for each subclade in the phylogeny. Subclade disparities are then standardized by total clade disparity to produce a measure of relative subclade disparity. To compute disparity through time, we move from root to tip, computing the average relative disparity at each node as the mean relative disparity of all clades with ancestral lineages alive at that time.
Low values of average subclade disparity indicate that, on average, individual clades alive at that time contain a small amount of total morphological variation, relative to the entire clade. Under the early burst scenario, average subclade disparity is expected to decline rapidly during the early part of clade history as lineages rapidly diverge into distinct adaptive zones. Later, disparity is expected to level off as divergence slows and subclades diversify within adaptive zones, leading to low disparity within deeply divergent subclades, relative to total clade disparity. How To Install Schluter Kerdi Waterproofing Membrane. Introduced the Morphological Disparity Index (MDI) as a means of testing whether the observed disparity through time curve differs from the expectation of a time-homogeneous Brownian motion process. Briefly, a large number of datasets are simulated under a BM model using the maximum likelihood estimate of the Brownian rate parameter and disparity through time curves computed for each simulation.
MDI is then computed as the area between the average curve from the simulated data and the curve for the observed data; this is in essence a form of parametric bootstrapping. A negative MDI, meaning that the majority of the area between the curves falls below the curve from the simulated data, indicates that subclades contain lower levels of disparity than predicted under a constant rates process, and supports the notion of a slowdown in rate. Presented a small modification of this approach wherein the area between the observed disparity curve and all simulated curves are computed and the proportion of cases in which the area between the curves ≤ 0, that is the proportion of cases in which the curve for the observed data falls below the simulated data, is taken as a probability that the MDI is significantly more negative than expected under a time-homogeneous BM model. The node height test () uses the relationship between the absolute magnitude of standardized independent contrasts () and the height above the root of the node at which they were computed to identify early bursts of trait evolution.
A significant, negative relationship between the two (i.e., larger contrasts occurring deeper in the tree) indicates that the rate of trait evolution had slowed through time. This method is similar to approaches used to evaluate the fit of a Brownian Motion model to trait data before performing a contrasts () or phylogenetic generalized least squares () analysis to test for an evolutionary correlation between quantitative characters. In the context of the node height test however, it is used to reject the null hypothesis of rate constancy through time, rather than to justify its use as an appropriate assumption (). Suggested using a randomization procedure to assess the significance of a node height test slope. To our knowledge, no simulation-based procedure has been utilized to evaluate whether the slope of a node-height test differs significantly from a null expectation.
The last approach is to use maximum likelihood (ML) to fit a model in which the rate of trait evolution is permitted to decrease through time (an Early Burst model; hereafter EB) and to compare this to a model of a trait evolving under a time-homogeneous BM using some model selection criterion such as a Likelihood Ratio Test (LRT), Akaike Information Criteria (AIC: ) or Bayesian Information Criteria (BIC: ). BM and EB are conceptually very similar in that they both assume that the trait values at the tips come from a multivariate normal distribution with the expected value for all tips equal to the state at the root of the tree. In fact all current univariate models of continuous trait evolution, including the OU process (;; ) and the commonly used “Pagel” models, ( λ, κ, δ;, ), can be generalized in the same form. Following the notation of, we denote X to be a column-vector of tip values and C to be a N × N matrix (where N is the number of tips) describing the phylogeny in terms of branch length such that C i,j is the distance from the root node to the most recent common ancestor of tips i and j. The (log) likelihood ℒ of the model can therefore be written as.
(2) meaning that the rate of evolution is constant across the clade and the variance accumulates proportional to the branch lengths. Under the “Accelerating Change/Decelerating Change” (AC/DC) model (), the rate of trait evolution is permitted to accelerate or decelerate exponentially as a function of time.
Note that we could also formulate a “linear change” model where the rate increases or decreases linearly with time. We limit our focus here to the exponential change model as it better fits the Simpsonian view of adaptive radiation as rapid early phenotypic diversification that subsequently slows as niches within adaptive zones become saturated. We follow and constrain the model such that the rate of change is only allowed to decrease towards the present and refer to this constrained form as the EB Model. If the starting rate of evolution is σ 0 2 and the parameter describing the rate at which σ 2 changes is denoted as r then. (3) Note that if r = 0, this model reduces to BM as σ 2 remains constant throughout the clade's history. Posterior Predictive Approach An alternative way to assess the fit of BM and EB models to comparative data is to simulate under both models and compare how well each does at predicting the observed distribution of trait values. If the observed data truly evolved under an EB-like process, then simulating under BM should do a very poor job of predicting them.
Simulating under EB, on the other hand, should result in trait values that are very similarly distributed. Both DTT and the node height test can readily be accommodated in such a posterior predictive framework as both produce a single summary statistic describing how phenotypic disparity changes through time. By generating a large number of summary statistics from data simulated under each process, we can compare our observed summary statistics to these predictive distributions and generate posterior predictive P values for each. In the case of an EB-like process, data simulated under BM should result in low posterior predictive P values (. We wrote a simple Markov chain Monte Carlo (MCMC) algorithm to sample model parameters for BM and EB from their posterior distributions, and to generate simulated datasets under the sampled values (). Our sampler followed other recent MCMC implementations applied to comparative datasets (e.g.,; ), so we will not give full details here.
R code to perform the analyses has been deposited at (doi:10.5061/dryad.6m2q0) and is incorporated in a forthcoming release of the geiger package (; Eastman, J., Pennell, M., Slater, G., Brown, J., FitzJohn, R., Alfaro, M., Harmon, L., Unpublished data) for R (R ). Briefly, at each step in the MCMC we proposed a new value for the model parameters, the Brownian rate (σ 2) and, in the case of the EB model, the exponential change parameter ( r). We used the restricted ML algorithm of to evaluate the likelihood, following equation 2.5 in, and accepted or rejected proposals based on the standard Metropolis–Hastings acceptance ratio.
We set a flat improper prior of on log(σ 2). Large negative values for the exponential change parameter of the EB model can cause singularity issues when attempting to invert the transformed phylogenetic variance-covariance matrix.
We therefore used a lower bound on the uniform prior on this parameter that was computed from the height, T, of the tree. Flow chart showing the steps involved in testing for early bursts of trait evolution in our simulated datasets.
See Materials and Methods for details on each analysis. Our MCMC differed in one significant respect from other implementations for comparative data.
In addition to sampling parameter values from the chain at each sampling step, we also generated a simulated dataset under the fitted model using the current model parameters (). We then computed the slope for the node height test performed on the simulated data and MDI between the observed and simulated data, recording these values to our output file along with sampled parameters.
We used the natural logarithm of the absolute value of standardized contrasts in our node height computations, rather than untransformed absolute values of standardized contrasts as in to ensure that we were testing for exponential declines in rate. At the end of each MCMC run, we obtained a sample of parameter values from the posterior distribution of the fitted model, as well as posterior predictive distributions for the node height test slope and MDI. We subsequently computed a posterior predictive P value for MDI by computing the quantile of the posterior predictive sample containing zero (that is, the quantile at which there is no difference between the observed and simulated data). We obtained the equivalent probability for the node height test by computing the proportion of cases in which the posterior predictive samples for the node height slope were less than or equal to the slope for the observed data.
Computing a posterior predictive P value in this way does not allow one to select a model as the best fitting in the way that AIC scores might. Rather expected values lying in the tails of the predictive distributions indicate poor predictive ability of the fitted model, while values falling close to the center of the distribution indicate that the model is better at predicting the observed data. Comparison of Method Performance. We performed a series of simulations to compare the power of our posterior predictive formulations of DTT and the node height test to that of the Maximum likelihood EB model.
We did not compare our posterior predictive versions to standard formulations of DTT and the node height test as the latter provide only a measure of whether or not an observed dataset fits with predictions of the EB model, rather than allowing comparisons of model adequacy. For each simulation, we generated a phylogenetic tree under a birth–death process with a speciation rate λ = 0.1 and an extinction rate μ = 0.09 using the R package TreeSim (). We conditioned simulations on 100 extant taxa, and subsequently rescaled the total depth of all trees to 10 time units. Preliminary simulations (data not shown) using a range of λ and μ values did not change our results and so we use the same parameters throughout this paper for the sake of consistency.
We then simulated trait evolution under both BM and EB models. For BM, variation in the rate of character evolution should not affect model fitting, as rate variation has no effect on phylogenetic signal in trait data (). Nevertheless, we confirmed this by simulating with σ 2 drawn from a range comprising (0.001, 0.1, 1, 10). For EB, choosing a meaningful range for r is difficult as the impact of variation in this parameter on the distribution of trait values and, subsequently, on our ability to detect the EB model is explicitly dependent on the age of the clade being examined; for a given value of r, as the age of a clade increases, the rate has more time to decay from its initial value and the resulting impact on the distribution of trait values among and within clades becomes more marked. For comparative purposes, it can be more useful to think of r in terms of its impact on the half-life of the rate, t 1/2 rather than in terms of the absolute magnitude of the parameter itself. For an exponentially decaying process occurring at rate r, the half-life is given.
In an attempt to improve on our ability to detect early bursts in the presence of outlier taxa, we made one modification to to our posterior predictive MCMC. In addition to using ordinary least squares regression to test for a relationship between contrast size and node height in the node height test, we also used a robust regression.
Robust regression approaches are designed to reduce the influence of outlying data points by identifying and down-weighting them, rather than removing them entirely. A robust regression procedure has been used for this very reason by when estimating changes in evolutionary rate relative to sampling interval length from paleontological data. We used a ML-type (M-estimation) robust regression procedure () implemented using the rlm function in the R package MASS ().
M-estimation procedures generate weights for each observation that are used to reduce the influence of outlier data on computation of the slope. Weights are estimated using an iteratively reweighted least squares procedure (), optimizing the equation. Where w i is the weight and ( y i – x ′ b) x i is the residual of the i-th observation. We used the Huber weighting scheme (), which applies weights of 1 to observations that do not deviate from the model predicted value (i.e., those with residuals ≈ 0).
Observations with larger residuals are down-weighted by applying weights 6 half-lives to have passed before the EB model was preferred on average with strong support ( >0.95;, b). Support for EB over BM increased with the number of elapsed half lives under both likelihood approaches, but the increase was more rapid for the likelihood ratio test ().
Mean support for EB based on Akaike Weights was lower than 0.5 when as many as 3 half-lives had elapsed. The two posterior predictive approaches performed reasonably. For EB with t 1/2 = ∞ (i.e., BM) mean posterior predictive P values for both were 0.5, as expected for a nested null model, and as the number of elapsed half-lives increased support for BM decreased ( and ).
At faster rate declines (number of elapsed half lives ≥ 9), all approaches performed comparably, although power for the MDI approach was lower than for the node height or likelihood ratio tests. Posterior predictive P values derived using simulation from the posterior distribution of parameters for the EB model followed expectation (), although weak bursts resulted in posterior predictive P values that were lower than the expected value of 0.5; this can be explained by an upper bound of 0 on our uniform prior for r. Mean P values rapidly converge on 0.5 as the number of elapsed half-lives increases. Distinguishing early bursts of trait evolution from Brownian motion in simulated datasets.
Support for EB is shown for elapsed rate half-lives (see text) ranging from 0 (i.e., BM) to 10. Support for EB is derived from a) mean posterior predictive P-values for Disparity Through Time and the Node Height Test when fitting and simulating under a BM model, and P-values from likelihood ratio tests comparing the fit of EB and BM models, and; b) median Akaike weights. Quantiles of predictive distributions derived under an EB model that contain the expected summary statistic value are shown in c). Here, values close to 0.5 indicate a close correspondence between simulated and observed data. Note that a) shows 1- P values and thus values ≥ 0.95 indicate low support for BM in favor of EB. Values are shown in this way to facilitate visual comparison with support under Akaike weights.
Distinguishing early bursts of trait evolution from Brownian motion in simulated datasets. Support for EB is shown for elapsed rate half-lives (see text) ranging from 0 (i.e., BM) to 10. Support for EB is derived from a) mean posterior predictive P-values for Disparity Through Time and the Node Height Test when fitting and simulating under a BM model, and P-values from likelihood ratio tests comparing the fit of EB and BM models, and; b) median Akaike weights.
Quantiles of predictive distributions derived under an EB model that contain the expected summary statistic value are shown in c). Here, values close to 0.5 indicate a close correspondence between simulated and observed data.
Note that a) shows 1- P values and thus values ≥ 0.95 indicate low support for BM in favor of EB. Values are shown in this way to facilitate visual comparison with support under Akaike weights. When BM was the generating model, variation in σ 2 had no effect on our ability to select among models, as expected and model selection perfomance was appropriate. Full details of these results are provided in the Supplementary Materials. Outlier Taxa and Robust Regression Adding convergent taxa had a substantial impact on our ability to detect an underlying early burst of trait evolution. When convergent taxa were added randomly through the phylogeny, Akaike weights for the EB model were low, even when only one or two convergent taxa were added (), even though the underlying burst was of a strength that should be detectable. The likelihood ratio test performed slightly better, but posterior predictive P values for MDI and, in particular, the node height test performed much better ().
We were on average able to strongly discount the null hypothesis of a BM process with up to 5% of taxa exhibiting phylogenetically random convergence using the posterior predictive node height test. These qualitative observations are confirmed by computation of power of the three approaches (). All three performed better for phylogenetically clustered convergence. On average, an underlying strong burst could be detected on the basis of Akaike weights with convergent clades of up to five taxa added and we could strongly discount the null BM model using MDI with clades of up to 10% convergence (). Again, the posterior predictive node height test out-performed the other approaches (), on average providing poor support for the null with convergent clades containing up to 20% of the total number of taxa added to our datasets (). The use of robust regression in place of ordinary least squares regression in the node height test resulted in a slight, but notable improvement in power () and decrease in support the null model for both types of convergence.
Using robust regression, we found poor support for the null BM model with up to 10% phylogenetically random convergent taxa (). For ordinary least squares regression this behavior dropped to tolerating only 5% convergent taxa. The greatest benefits again occurred for phylogenetically clustered convergence. Here, we found, on average, poor support for BM with up to 30% convergent taxa. For OLS, this figure dropped to ∼ 20 convergent taxa (). Comparison of median performance of the node height test using least squares and robust regression with a) phylogenetically random b) and phylogenetically clustered convergence. Dashed lines indicate 95% quantiles.
Analysis of Cetacean Body Size Evolution We first used ML to fit three models to our cetacean body length data: a time-homogenous BM model, an EB model, and the mysticete-shift model that allowed for elevated rates in the stem mysticete lineage. ML parameter estimates for the early burst model () suggest declining rates of body length evolution with an initial rate twice that estimated under BM and an exponential decline parameter of −0.023, equivalent to a rate half-life of 30.14 million years.
For the mysticete-shift model, parameter estimates indicate that a rate increase of 12.5 × is required along the branch leading to crown mysticetes to explain the distribution of extant cetacean body sizes. However, Akaike weights demonstrate equivocal support for all three models (). Brownian motion is the best fitting model, but receives only 41% of the Akaike weight and, while the other two models receive slightly less weight, they cannot be ruled out.
Although the EB model receives the lowest support among the candidate models, based on ML parameter estimates for this model and the crown age of cetaceans in our phylogeny, only 1.22 rate half-lives would have passed over the duration of cetacean evolution. Taken in conjunction with our simulation results (), this finding suggests that even if cetaceans did undergo an early burst of body size evolution, we should not expect to find support for this model using ML with a dataset comprising extant taxa only. Posterior predictive simulations reveal a slightly clearer picture of body size evolution in cetaceans. Predictive distributions for MDI under all three candidate models are left-shifted (), indicating that the observed data show a greater degree of among-clade partitioning of phenotypic variation than is expected from the fitted models. This is particularly accentuated for the time-homogeneous BM model (), where the expected value of 0 falls at the 95th percentile of the predictive distribution. The EB and mysticete-shift simulations provide a closer fit, although the expected value of zero falls between the 75th and 80th percentiles for both. Visual inspection of the predictive distributions (Fig.
6b,c) further suggests that the mysticete-shift model and the EB model are a much closer fit to the data than the homogeneous BM process, an observation confirmed by location statistics for the three distributions (). Posterior predictive distributions of MDI for cetacean body length data generated under a) BM b) EB, and c) Mysticete shift models.
Dashed verticle lines indicate the expected value of 0. The node height test using ordinary least squares regression shows a negative relationship between the ln(absolute value of contrasts) and node heights, although this relationship is not significance at α = 0.05 (β ols = −0.046, p = 0.051). Posterior predictive distributions of node height test slopes support the results from ML and MDI; all models appear to be poor predictors of the relationship between contrast size and node height, which is more negative than expected from the candidate model pool ( and ). The use of a robust regression estimator of the slope alters this finding substantially. Huber weights for 12 contrasts were.
Cetacean phylogeny used in our analyses. Shaded node labels indicate nodes that were down-weighted in computation of the robust regression slope. Lighter shades indicate nodes that were more heavily down-weighted. DISCUSSION Evolutionary biologists have increasingly come to rely on ML or, more recently, Bayesian inference for assessing the fit of evolutionary models to quantitative trait data. The typical work-flow in a macroevolutionary study involves fitting a series of models using ML and then comparing the fit of the candidate pool to the data using likelihood ratio tests or information theoretic criteria (). If AIC is used, the model with the lowest AIC score or highest Akaike weight is then declared the winner, and inferences are subsequently drawn about the tempo and mode of trait evolution in the clade being investigated.
These approaches have many desirable properties. In particular, model selection using information theoretic approaches allows users to simultaneously compare a pool of candidate models rather than performing a series of pairwise comparisons. Furthermore, because informatic theoretic approaches do not require a model describing the null expectation, selection of a “best” model does not imply that model is correct but rather than it comes closest to describing the underlying data. However, these approaches, and indeed their advantages, also lead to limitations.
For example, a given model might receive the highest weight of the candidate pool but simulating from it might fail to produce anything like the observed data. In this case, the winning model does not adequately describe the underlying evolutionary process in the clade being studied, but how are we to know this?
Alternatively, we might find that we cannot differentiate among candidate models using information theoretic criteria. It is possible in this case however that our inability to select a winning model is not driven by the lack of a good model in our candidate pool, but rather by one or two data points that deviate from the ancestral pattern and cloud our ability to select the true model. Model fitting alone does not tell us however if this is the case. In this article, we have demonstrated that posterior predictive approaches have the potential to greatly improve our ability to distinguish among modes of trait evolution in comparative data.
We found that by using posterior predictive P values derived from the morphological disparity index () and, in particular, the node height test slope (), we recovered strong support for the EB model from datasets simulated under moderately strong early bursts that, in some cases, could not be detected using information theoretic approaches. This unexpected result is appealing for two main reasons. First, the “Early Burst” model is simply difficult to detect with phylogenetic comparative data (), and any method that increase our power to do so is helpful. Interestingly, the patterns we have found regarding the power to detect slowdowns in trait evolution are, in some ways, exactly the opposite to the findings of studies that have investigated slowdowns in lineage diversification rate (; ).
For example, simulated phylogenies under time-varying diversification rates and found that the power to detect early bursts was greatest early on and that the signal was subsequently erased by extinction later in the clade's history (see also,; ). Here, we demonstrated that under a model of declining trait evolution, we expect the signal to actually get stronger through time. This finding supports the notion that, if adaptive radiations are characterized by early bursts of both lineage and trait diversificaiton, the signal for the early burst may be better retained in trait data (). Although found little support for EB-like processes in their large number of comparative datasets, several authors have found support for decelerating rates of evolution in individual clades (;;;;; ).
It is notable that none of these studies used the ML formulation of the EB model to do so; in each case their findings were based on DTT, the node height test, or both. () did use ML but not the standard EB model; we refer readers to their paper for details).
It is possible that these methods are simply more forgiving of noisy data than the analytical approach of, although more work is clearly required to fully understand this phenomenon. The second appealing aspect of posterior predictive approaches is that they provide a built-in check of model adequacy.
By simultaneously fitting models and simulating from their parameter posterior distributions, we are not only able to compare model fit, but also to ask how close each comes to predicting the distribution of data observed in the focal clade. A word of caution is warranted here: The summary statistics that we used to compare EB and BM are useful for comparing time-homogeneous rate processes to those in which rates can vary through time because they use estimates of the rates along branches or the expected disparity resulting from the evolutionary process. The metrics are less suited to processes in which the expected value of a trait changes, such as an evolutionary trend (;, ) or a multi-peak OU process (; ). Although this means that MDI and the node height test slope are unlikely to be useful for assessing the fit of these kinds of models, alternative summary statistics could easily be derived to do this. To the best of our knowledge, ours is the first study investigating the influence of “outlier” taxa on model selection and fit. The implicit assumption in fitting and comparing models of trait evolution, that patterns should be homogeneous across the clade, is clearly unrealistic and it is alarming that even a small number of outlier taxa had such a strong effect on our ability to infer the true model.
This is especially relevant for tests of early bursts in the context of adaptive radiations as there is no reason why we would expect a priori that an entire clade should show an early burst pattern, even under the simple scenario described. Some lineages may escape the ancestral adaptive zone, perhaps by moving to a new geographic region or evolving a novel key innovation ().
A number of approaches have been developed to investigate rate heterogeneity in rates of trait evolution across the tree (;;;; ) but these have so far, been restricted to the case of multiple rate BM. We took a slightly different approach here. Rather than attempting to identify exceptional lineages, we sought to look for general patterns—that is to pull a broad signal out of the noise. As the node-height test involves fitting a linear-regression model to the data, it is natural to turn to established methods from the statistics literature, such as robust regression (), to down-weight the contribution of “outlier” taxa in the test. In applying our posterior predictive robust regression approach to the cetacean dataset, we were able to show that a constant rates process and a process allowing rapid evolution in the stem mysticete lineage were poor predictors of extant cetacean body lengths. The early burst model is a better, although not perfect fit to these data. Recovered a similar result but were forced to arbitrarily remove two contrasts that they had visually identified as outliers in their node height test regression.
Our use of a robust regression identified these outliers, as well as several additional influential contrasts, and allowed us to appropriately weight them when computation of the node height test slope. In the process, we removed the arbitrariness of visually identifying outliers and avoided removing them altogether.
It makes sense to ask whether the contrasts identified and downweighted by the robust regression procedure make biological sense. The two nodes identified in were recovered here as possessing large, positive residuals consistent with interpretation in that paper. The contrast between the blue whale Balaenoptera musculus and a clade of other roquals also generated a positive residual that was downweighted here, perhaps suggested accelerated evolution in the lineage leading to the largest animal to have ever lived. All other downweighted contrasts possessed negative residuals.
The two most heavily downweighted nodes were for pairs of recently diverged sister species that do not differ in body length, while the other node to receive a weight of.